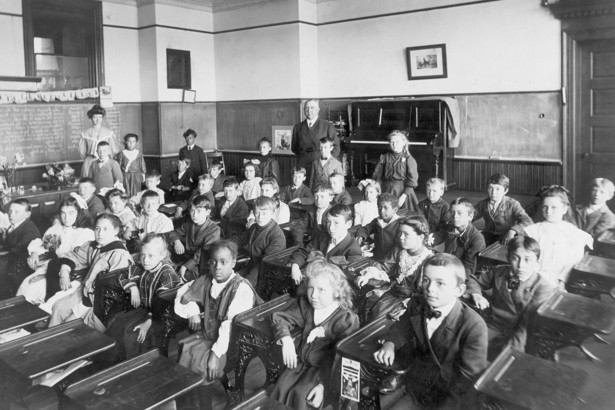
The first “teaching machine” was invented nearly a century ago by Sydney Pressey, a psychologist at Ohio University, out of spare typewriter parts. The device was simple, presenting the user with a multiple-choice question and a set of answers. In “teach mode,” the machine would advance to the next question only once the user chose the correct answer. Pressey declared that his invention marked the beginning of “the industrial revolution in education”—but despite his grand claims, the teaching machine failed to gain much attention, and soon faded into obscurity.
It stayed there until the 1950s, when the famed behaviorist B.F. Skinner introduced a teaching machine of his own (Skinner blamed “cultural inertia” for Pressey’s previous lack of success). His new device taught by showing students questions one at a time, with the idea that the user would be rewarded for each right answer.
This time, there was no “cultural inertia.” Teaching machines flooded the market, and backlash soon followed. Kurt Vonnegut called the machines “playthings” and argued that they couldn’t prepare a kid for “one-millionth of what is going to hit him in the teeth, ready or not.” Fortune ran a story headlined “Can People Be Taught Like Pigeons?” By the end of the ‘60s, teaching machines had once again fallen out of favor. The concept briefly resurfaced again in the ‘80s, but the lack of quality educational software—and the public’s perception of mechanized teachers as something vaguely Orwellian—meant they once again failed to gain much traction.
But now, they’re back for another try.
Scientists in Germany, Turkey, the Netherlands, and the U.K. are currently working on language-teaching machines more complex than anything Pressley or Skinner dreamed up. These devices will help students learn basic vocabulary and simple stories, using microphones to listen, cameras to watch, and artificial neural networks that will analyze all the information that’s collected. The machines are part of L2TOR (pronounced “El Tutor”), a program funded by the European Union to develop artificially intelligent teachers for preschool-aged children.
But the machines won’t only teach and collect data on their students’ language skills—they’ll also monitor things like joy, sadness, boredom, and confusion. Human teachers can see and hear their students and make sense of all nonverbal cues they get from the class; these machines are being designed to do the same.
“The problem with previous generations of teaching machines was their complete lack of social intelligence,” says Stefan Kopp, an artificial-intelligence researcher at Bielefeld University in Germany and one of the scientists working on L2TOR. “Yet it’s possible to design emphatic machines. Our robots will notice tears, smiles, frowns, yawns … and dynamically adjust to how a child feels.” Past research has shown that “affect-sensitive” teaching systems, as they’re known, may be more effective at imparting knowledge than machines that don’t take emotions and experience into account.
The L2TOR researchers who launched their project earlier this month, still have a few years before they can measure their technology against human educators, but similar projects have offered some hints about potential challenges. FACET, a commercially available image-processing software that analyzes 19 different facial-muscle movements, works with nearly 80 percent accuracy. Earlier this year, a research team at the University of Notre Dame used it to identify children’s boredom, confusion, and delight as they played educational games, using videos taken with laptop cameras in real classrooms. In more than one-third of instances, FACET recognized nothing at all. Kids wriggled, covered their faces with their hands, talked with their friends—all sorts of things, except for sitting still in front of the cameras.
And successfully interpreting students’ emotions is just one challenge; knowing how to react to that information is another. What should a robot do with a 5-year-old who is frustrated, or bored, or has just thrown a paper airplane right into its robotic face?
To figure out how to imbue their machines with human-like reaction skills, Kopp and his colleagues plan to spend some time in kindergarten classrooms, observing the teachers at work. “We need to learn more about their methods, learn from their experience, and then program our robots to act like them,” Kopp says. “We want the machines to be as friendly to kids as possible, yet I think a robot should react to bad behavior.” The challenge is figuring out how these machines can exert authority in a way that teaches the kids how to behave, in addition to the lessons of the day.
Another thing that remains to be seen: whether the kids can learn to relate to the machines the way they would ordinary teachers. “People, especially children, tend to ascribe human qualities to objects—teddy bears and so on. We also know that part of the brain responsible for our interpersonal skills becomes active in the presence of social robots. Yet, adults who took part in these experiments knew that they were dealing with machines, with objects,” Kopp says. “But nobody has ever tried such a thing with 5-year-olds. We can’t tell if these kids will treat robot tutors like toys or like living, caring persons.”